Transforming Business with Machine Learning Annotation Tools
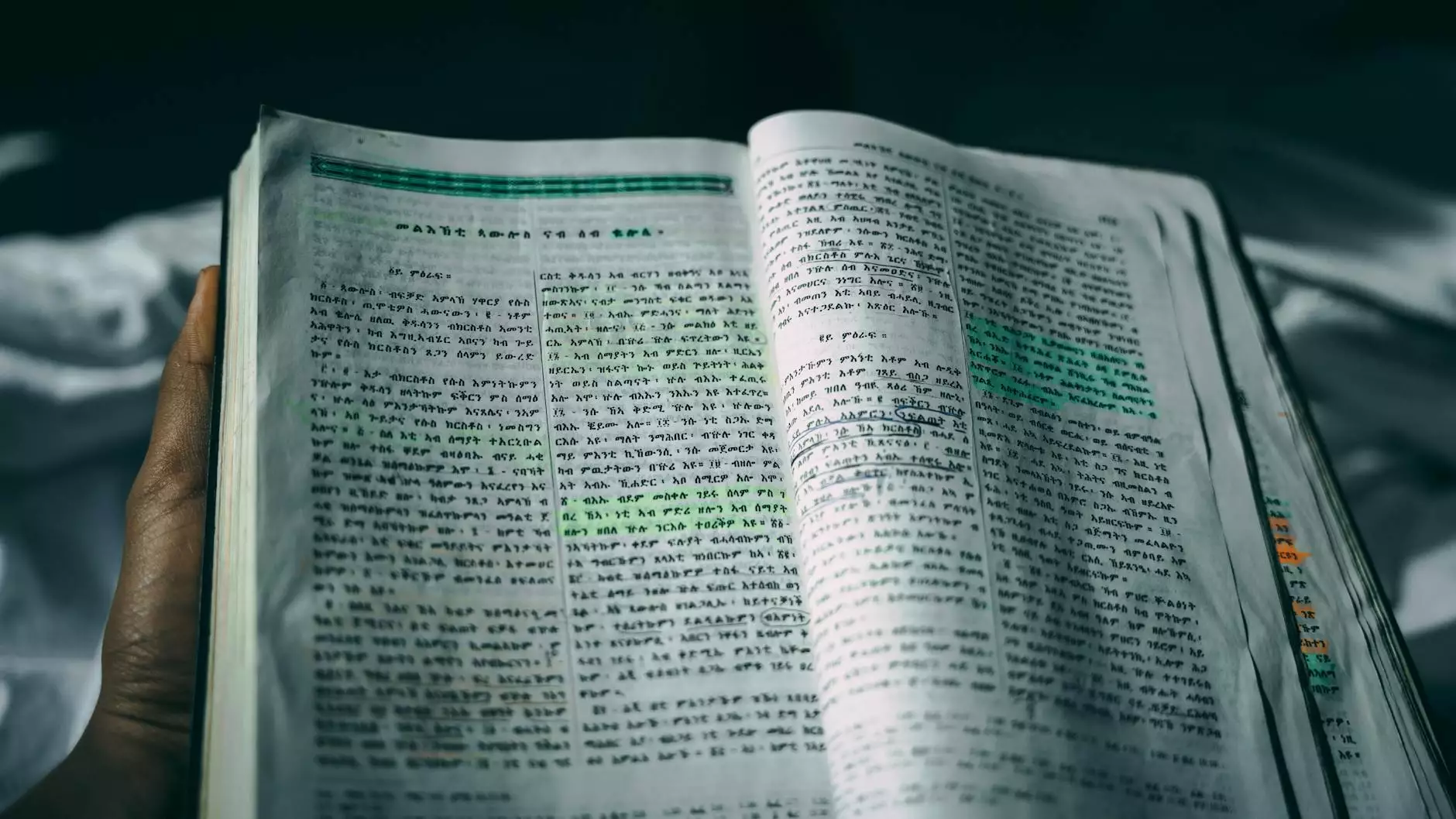
The rapid evolution of technology in recent years has fundamentally changed the business landscape. One of the most significant advancements has been the emergence of machine learning (ML), which has enabled businesses to analyze vast amounts of data and make informed, strategic decisions. Central to this process is the use of machine learning annotation tools, which play a crucial role in preparing data for training machine learning models. This article delves into the importance of these tools and how they can transform your business operations.
The Role of Machine Learning in Modern Business
Machine learning has become an indispensable asset, particularly in the fields of software development, marketing, finance, and customer service. By automating decision-making processes and deriving insights from data, machine learning enables companies to:
- Enhance Operational Efficiency: Streamlining operations and reducing manual efforts.
- Improve Decision-Making: Making data-driven choices based on accurate predictions.
- Boost Customer Experiences: Delivering personalized services based on customer behavior analysis.
- Drive Innovation: Creating new products and services based on predictive analytics.
Understanding Machine Learning Annotation Tools
At the heart of effective machine learning is high-quality data. Machine learning annotation tools are designed to preprocess this data, making it usable for training algorithms. These tools provide a way to label and categorize data accurately, which is essential for tasks such as image recognition, natural language processing, and more. Here’s a deeper look at their functionalities:
Types of Data Annotation
Machine learning annotation can be applied to various types of data, including:
- Text Annotation: Involves tagging parts of text data for sentiment analysis, keyword extraction, etc.
- Image Annotation: Used in computer vision tasks to identify objects, people, or actions in images.
- Audio Annotation: Involves labeling audio data for speech recognition and classification tasks.
- Video Annotation: Tags objects and activities within a video for tasks like action detection.
Key Features of Machine Learning Annotation Tools
When considering machine learning annotation tools, businesses should look for:
- User-Friendly Interfaces: Intuitive design that makes it easy for teams to annotate without extensive training.
- Collaboration Tools: Features that allow teams to work together seamlessly.
- Quality Control Mechanisms: Ensuring data accuracy through validation and review processes.
- Integration Capabilities: Compatibility with existing software and data management systems.
- Scalability: Ability to handle increasing datasets as the project grows.
Why Your Business Needs Machine Learning Annotation Tools
Incorporating machine learning annotation tools into your workflow can yield numerous benefits:
1. Enhanced Data Quality
Good quality data is essential for effective machine learning. Machine learning annotation tools help improve data quality through systematic labeling, which reduces errors and increases the reliability of outputs.
2. Increased Efficiency
Manual annotation is time-consuming and prone to errors. Automated and semi-automated annotation tools can expedite the data preparation phase, allowing your team to focus on analysis and modeling rather than data entry.
3. Cost-Effectiveness
Investing in machine learning annotation tools can significantly cut costs in the long run. By minimizing human resources for data annotation and reducing the time to market for new products, businesses can achieve a better ROI.
4. Competitive Advantage
Utilizing high-quality annotated data allows businesses to develop superior machine learning models, which can lead to better insights, customer experiences, and ultimately, a stronger market presence.
Best Practices for Using Machine Learning Annotation Tools
To maximize the benefits of machine learning annotation tools, consider the following best practices:
1. Define Clear Annotation Guidelines
Establish a robust set of guidelines for annotators. This ensures consistency, which is vital for maintaining the integrity of your data.
2. Monitor Data Quality Regularly
Use built-in quality control features to regularly review and validate annotated data. This helps in identifying any lapses in quality and allows for immediate corrective measures.
3. Provide Continuous Training
Regularly train your team on the latest tools and update them on project-specific requirements. This empowers them to produce more accurate annotations.
4. Leverage Automation
Utilize automated features of the annotation tools to reduce manual workloads and enhance productivity. Tools that incorporate AI for pre-annotation can significantly speed up the process.
Implementing Machine Learning Annotation Tools in Your Business
Integrating these tools into your software development workflow requires a strategic approach:
1. Assess Your Needs
Evaluate the specific requirements of your projects. Different tasks may require different types of annotation, so understanding your needs is crucial.
2. Choose the Right Tool
Research and select a machine learning annotation tool that aligns with your business goals, offers scalability, and fits within your budget.
3. Pilot Testing
Before a full-scale implementation, conduct pilot tests to identify any potential issues and gather feedback from users.
4. Full Implementation
Once satisfied with the pilot results, proceed with a complete rollout, ensuring that all teams are adequately trained and supported throughout the transition.
Future Trends in Machine Learning Annotation
The future of machine learning annotation tools looks promising, with several trends emerging:
1. Increased Use of AI for Annotation
Artificial intelligence will continue to play a pivotal role in automating data annotation, alleviating the workload on human annotators.
2. More Collaborative Tools
As remote work becomes the norm, tools that support collaborative annotation will see increased adoption, enhancing teamwork across geographies.
3. Focus on Ethical Data Use
With growing concerns about data privacy and ethical AI, businesses will prioritize annotation practices that are transparent and compliant with regulations.
Conclusion
Machine learning annotation tools are essential for any business looking to leverage the power of machine learning effectively. By providing high-quality data, enhancing operational efficiency, and enabling better decision-making, these tools not only improve the quality of AI models but also position businesses to thrive in an increasingly competitive landscape.
As you consider implementing these tools, align them with your strategic objectives, invest in training and development, and stay informed on industry trends to continuously optimize your approach. Ultimately, the right machine learning annotation tools will empower your business to unlock significant insights, drive innovation, and achieve sustainable growth.