The Importance of Machine Learning Data Annotation in Today's Business Landscape
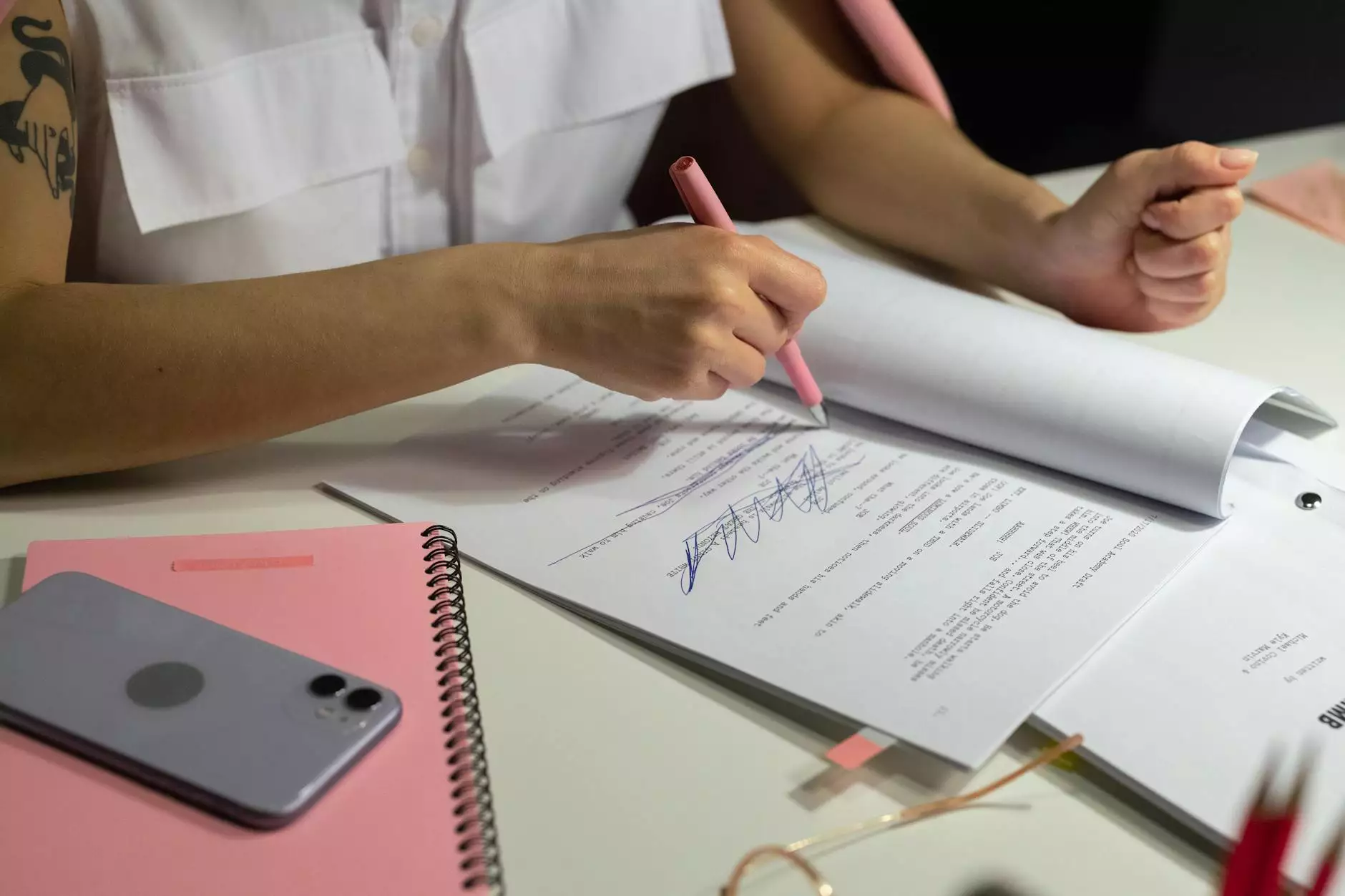
In the rapidly evolving world of technology, machine learning has emerged as a game changer across various industries. However, its success largely hinges on one crucial element: data annotation. In this article, we will delve into the intricate relationship between machine learning data annotation and business performance, particularly in the realm of Home Services and Keys & Locksmiths.
Understanding Machine Learning Data Annotation
At its core, machine learning data annotation is the process of labeling data to make it understandable for machine learning algorithms. This involves categorizing the data into formats that machines can read and learn from effectively. For businesses, this means transforming raw data into valuable insights.
Why Is Data Annotation Essential?
The importance of data annotation in machine learning cannot be overstated. Here are several reasons why:
- Improved Accuracy: Annotated data helps machine learning models predict outcomes more accurately.
- Enhanced Learning: Quality annotations lead to better training and more efficient models.
- Domain-Specific Applications: Tailoring data annotation to specific industries increases model relevance and effectiveness.
- Scalability: Well-annotated data can be scaled for various applications, increasing adaptability in different business scenarios.
Machine Learning Data Annotation in Home Services
In the Home Services sector, machine learning data annotation plays a pivotal role in refining customer experience and improving operational efficiency. Let’s explore how:
Customer Interaction Analysis
By annotating customer interaction data, businesses can analyze common queries and service trends. This provides insights into customer behavior and preferences, allowing for:
- Personalized Marketing: Targeted promotions based on customer needs and historical data.
- Service Optimization: Adjusting service offerings based on demand trends.
Enhanced Decision-Making
Annotated data aids in making informed decisions regarding resource allocation. By analyzing data related to service requests and timeframes, businesses can:
- Optimize Scheduling: Efficiently managing technician schedules and reducing waiting times.
- Inventory Management: Understanding which services are in demand helps to maintain optimum stock levels.
Machine Learning Data Annotation for Locksmiths
The locksmith industry is becoming increasingly reliant on technology. Here, machine learning data annotation can greatly enhance service delivery and security measures.
Fraud Detection and Prevention
By annotating data from previous transactions and service requests, locksmiths can identify patterns indicative of fraud. This helps in implementing robust security measures such as:
- Real-Time Monitoring: Monitoring service requests in real-time to detect suspicious patterns.
- Risk Assessment: Evaluating the risk levels of various types of jobs based on historical data.
Inventory Management
For locksmiths, understanding which products and services are most requested can greatly impact inventory decisions. Annotated data helps in:
- Demand Forecasting: Anticipating the need for specific locks, keys, and related products.
- Targeted Promotions: Strategically marketing products based on customer demand analysis.
The Process of Machine Learning Data Annotation
Understanding how data annotation is performed is crucial for businesses looking to harness its benefits. The process typically involves several steps:
- Data Collection: Gathering raw data from various sources such as customer interactions, transaction logs, and service feedback.
- Data Cleaning: Removing irrelevant or erroneous data to enhance quality.
- Annotation: Expert annotators label the data according to predefined criteria. This could involve classifying customer inquiries, tagging service types, etc.
- Verification: Ensuring that the annotations are accurate and reliable, often through a second layer of review.
- Model Training: Feeding the annotated data to machine learning models for training.
Challenges in Machine Learning Data Annotation
While the benefits are clear, businesses must also navigate various challenges associated with machine learning data annotation. Some of these challenges include:
- Quality Consistency: Maintaining a consistent quality of annotations over time.
- Resource Intensive: The process can be labor-intensive, requiring skilled annotators.
- Rapid Changes in Trends: Data can quickly become outdated, necessitating continuous updates to annotation standards.
Enhancing Business Performance Through Data Annotation
The application of machine learning data annotation is a robust strategy for enhancing overall business performance. Here are key areas where businesses can leverage these insights:
Predictive Analytics
Businesses can utilize annotated data for predictive analytics, allowing them to forecast trends and make proactive decisions. This is particularly effective in:
- Customer Retention: Identifying customers who may churn and implementing strategies to retain them.
- Service Expansion: Predicting new service areas based on emerging customer demands.
Marketing Strategy Refinement
Data annotation helps businesses refine their marketing strategies by pinpointing effective campaigns and customer segments. This can lead to:
- Higher Conversion Rates: Targeting potential customers more effectively results in better sales outcomes.
- Brand Loyalty: Understanding customer preferences builds stronger relationships and fosters loyalty.
Conclusion: The Future of Machine Learning Data Annotation in Business
As industries continue to embrace technological advancements, the role of machine learning data annotation will only grow in significance. Businesses in the Home Services and Keys & Locksmiths sectors that invest in quality data annotation will undoubtedly reap substantial benefits, including improved efficiency, enhanced customer experiences, and increased profitability.
In conclusion, the integration of machine learning data annotation into business processes is not just a trend; it is a fundamental shift towards a more data-driven and customer-centric approach. Embracing this technology is essential for businesses eager to maintain a competitive edge in an increasingly digital world.